
Source, Sum of Squares, Degrees of Freedom, Mean.Three-Way Independent Samples ANOVA Done With SPSS. Define main effect, simple effect, interaction, and marginal meanThe most common type is type III which is returned by default in Stata, SAS, and SPSS. An example Imagine a clinical psychologist wanted to see the effects of a new antidepressant drug called Cheerup. However, SPSS can do these contrasts if you define them using syntax. Unlike one-way ANOVA, there is no way in factorial designs to define contrast codes through the Windows dialog boxes. Be done using SPSS’s built-in contrasts.

However,It is more efficient to conduct one study that includes both independentVariables. One approach could have been to conductTwo separate studies, one with each independent variable. Two independentVariables were investigated: (1) whether the companion was obese or ofTypical weight and (2) whether the companion was a girlfriendOr just an acquaintance. Compute the source of variation and df for each effect in a factorialStudy, the researchers were interested in whether the weight ofA companion of a job applicant would affect judgments of a maleApplicant's qualifications for a job. State the relationship between simple effects and interaction
Weight: Are applicants judged differently depending on the If so,There would be an interaction between the obesity factor andThere are three effects of interest in this Perhaps there is morePrejudice against a person with an obese companion if the companionIs a girlfriend than if she is just an acquaintance. For example, it is possible thatThe effect of having an obese companion would differ dependingOn the relationship to the companion. There is an interaction when the effect of oneVariable differs depending on the level of a second variable.
The main effect of WeightIs based on a comparison of these two marginal means. Similarly, the marginal mean for the level "Typical" is theMean of 6.19 and 6.59, which is 6.39. For example,The marginal mean for the level "Obese" is the meanThat this marginal mean is equal to the mean of 5.65 and 6.15,Which is 5.90. A marginal mean for a level of a variable is theMean of the means of all levels of the other variable. A main effect of an independent variable is theEffect of the variable averaging over the levels of the other variable(s).It is convenient to talk about main effects in terms of marginalMeans. Weight x Relationship Interaction: Does the effect of weightDiffer depending on the relationship with the companion?The first two effects (Weight and Relationship) are both mainEffects.
As shown below,These simple effects are not significantly different.The important questions are not whether thereAre main effects and interactions in the sample data. In thisExample, the simple effects of weight are 0.54 and 0.44. The difference is 6.59-6.15 = 0.44.Recall that there is an interaction when the effectOf one variable differs depending on the level of another variable.Is an interaction when the simple effects differ. Similarly, the simple effectOf Weight at the level of "Acquaintance" isBetween the "Acquaintance Typical" and the "AcquaintanceObese" conditions. TheDifference is 6.19-5.65 = 0.54. The simpleEffect of Weight at the level of "Girlfriend" isThe difference between the "Girlfriend Typical" and the "Girlfriend Obese" conditions.
The degrees of freedom for a main effect is always equal to the number of levels of the variable minus one. ANOVA tests main effects and interactions for significance.An ANOVA Summary Table for these data is shown in Table 2.Consider first the effect of "Weight." The degrees of freedom (df) for "Weight" is 1. This is where Analysis of Variance comesIn.
For the effect of "Weight," F = 10.4673/1.6844 = 6.214. The F ratio is computed by dividing the MS for the effect by the MS for error (MSE). The mean square (MS) is the sum of squares divided by the df. We skip the calculation of the sum of squares (SSQ) not because it is difficult, but because it is so much easier to rely on computer programs to compute it.
Therefore, these data provide no evidence for an interaction. The p value for the interaction is 0.8043, which is the probability of getting an interaction as big or bigger than the one obtained in the experiment if there were no interaction in the population. For the "Weight x Relation" interaction (W x R), the df = 1 since both Weight and Relation have one df: 1 x 1 = 1. The p value is 0.0136 and therefore the null hypothesis of noThe conclusion is that being accompanied by an obese companion lowers judgments of qualifications.The effect "Relation" is interpreted the same way.The conclusion is that being accompanied by a girlfriend leadsTo lower ratings than being accompanied by an acquaintance.The df for an interaction is the product of the df's of variables in the interaction.
It is also equal to the number of observations minus 1, or 176 - 1 = 175. Sample Sizes for All Four Conditions.The final row in the ANOVA Summary Table is "Total." The degrees of freedom total is equal to the sum of all degrees of freedom. Since there are four groups, dfe = 176 - 4 = 172.Table 3. The total number of observations is 40 + 42 + 40 + 54 = 176. The sample sizes of the four conditions in this experiment are shown in Table 3. In other words, you do not accept the null hypothesis just because you do not reject it.For "Error," the degrees of freedom is equal to the total number of observations minus the total number of groups.
Second, one of the independent variables is on the X-axis. It is important to consider the components of this plot carefully.First, the dependent variable is on the Y-axis. The reasons for this are complex and are discussed in the section Unequal Sample Sizes.Although the plot shown in Figure 1 illustratesThe main effects as well as the interaction (or lack of an interaction), it is called an interactionPlot. However, when there are unequal sample sizes, as there are here, this will not generally be true.
NonparallelLines indicate interaction. Notice that the two linesIn the graph are not parallel. By contrast, for subjects low in self-esteem, failure led to more attribution to oneself than did success. Interaction Plot for Made-Up Data.Clearly the effect of "Outcome" is different for the two levels of "Esteem": For subjects high in self-esteem, failure led to less attribution to oneself than did success. It is better to label the lines right on the graph, as shown here, than with a legend.Figure 5.
The dependent variable, Breaking Torque, was measured in foot-pounds. Table 6 shows the analysis ofA study described by Franklin and Cooley investigating three factorsOn the strength of industrial fans: (1) Hole Shape (Hex or Round), (2) Assembly Method (Staked or Spun), and (3) Barrel Surface (Knurled or Smooth). Lines do not have toThree-factor designs are analyzed in much theSame way as two-factor designs.
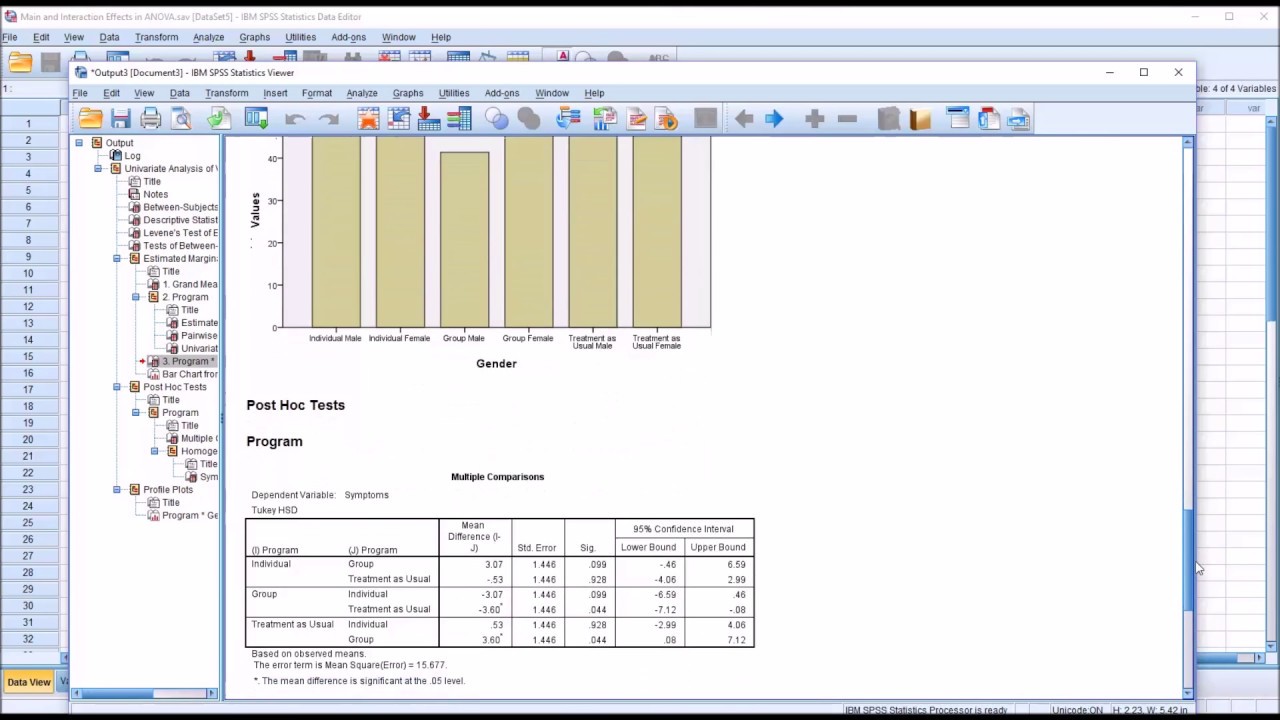
ANOVA Summary Table for Fan Data.
